With the breakthrough of artificial intelligence (AI) systems in modern life, there has been increasing pressure to incorporate this technology into medicine and healthcare.1 Fields such as radiology, emergency medicine, and telehealth have made significant strides toward implementing AI into diagnostic modalities and treatment algorithms, but often at the cost of medical ethics.2 An automated self-intelligent system cannot understand important concepts such as patient consent, data privacy, and implicit bias associated with the healthcare field. In this article, we explore these sensitive issues that will become more prevalent as the healthcare industry continues to adopt AI-based modalities.
Unraveling the bias in algorithms
AI has been employed in multiple studies in medicine to uncover hidden disease patterns within highly diverse clinical datasets. AI models can be used to identify, characterize, and predict diseases, which could potentially alter the path of severe illnesses.3 All AI systems rely heavily on the historical data fed into the initial algorithm to generate new protocols and methodologies.4 As a result, inherent biases in this data can become more pronounced, leading to flawed results.
For example, historical data suggests that in the healthcare system, patients from the LGBTQIA+ community, as well as patients from certain ethnic and racial communities, experience a high rate of disparity.5,6 Implicit issues such as racial profiling, gender disparities, etc., have often permeated the healthcare community. Incorporating this data may lead to the AI system exaggerating these biases and worsening medical outcomes. If these biases are considered during the initial implementation, AI can improve outcomes much quicker than traditional methods. Disparities in healthcare have garnered increasing attention in the last few years, and the use of AI can have broad implications for the overall quality of care.
AI algorithm biases are not reported well in peer-reviewed literature. A study published by du Toit et al. has noted that out of 63 articles reviewed on hypertension and evaluated against the Harmonious Understanding of Machine Learning Analytics Network (HUMANE) checklist, none of them addressed the algorithm’s bias, and a mere 10% mentioned it as a risk.7 The current AI and machine-learning literature has also not addressed this gap in other specialties. Therefore, healthcare and AI professionals must develop strict measures to recognize and rectify such algorithm biases. Ensuring that AI provides non-discriminatory and fair results across a diverse set of patients requires continued monitoring and validation. As the number of practicing physicians older than age 65 has grown in the last few decades, it is vital to ensure transparency and simplicity in algorithm decision-making to ensure improved uptake among this demographic.8 Also, implementing checklists like HUMANE fosters a culture of critical thinking and proactively mitigates bias. This increases the quality of academic papers and ensures the responsible development of AI that benefits everyone.
Empowering patients through informed consent
Patient autonomy is the cornerstone of medical ethics and has become even more critical in the age of AI.9 Older patients with multiple chronic health conditions face unique challenges.10 Many of these patients are skeptical of computer and AI-based modalities and reject them outright.11 An ethical system ensures that these patients are educated about the benefits of implementing AI tools before initiation. There should also be an option to opt out of these modalities if patients choose. Such a transparent process increases trust as patients have options when deciding on their healthcare needs. Many patients are concerned about the security and privacy of their healthcare information, and any educational material must address this vital issue to assuage potential concerns.
Responsible deployment of AI technologies
Regulatory bodies like the U.S. Food and Drug Administration (FDA) are crucial in the regulatory framework for AI applications.12 The regulatory landscape for AI is dynamic, and it is essential to implement safeguards, establish guidelines for ethical AI development, and prevent misuse. A comprehensive framework must be used to evaluate the overall impact on healthcare systems and our society. As patient welfare is always a priority, clear guidelines are necessary to ensure that AI applications meet strict ethical criteria. Various organizations use different data-gathering systems, which might also create roadblocks in operational activity and standardization.13 Additionally, frequent assessments and audits of AI systems can help maintain transparency and accountability.
Liability concerns
Liability concerns, particularly in adverse outcomes, present a complex challenge. While the primary responsibility for due diligence in the selection and application of AI technologies falls upon physicians and healthcare practitioners, the manufacturers and developers of these AI systems must also acknowledge and embrace their role in ensuring the safety and efficacy of their products. The emergence of AI-specific liability insurance offers a novel solution to manage malpractice claims that may arise from integrating AI in healthcare, reflecting the evolving landscape of medical legalities and responsibilities in the era of advanced technology.14
Hallucinations
Additionally, the implementation of AI in healthcare must address the issue of AI hallucination or AI misinformation, which can further complicate effective deployment. AI misinformation refers to AI-generated content that is not based on real data but is produced by extrapolating existing training data by the algorithm.15 Healthcare providers need to be mindful of this problem and exercise caution while acknowledging that AI cannot replace the individualized and personalized care that healthcare practitioners deliver.
Lastly, clinicians must be equipped with the skill sets required to navigate the ethical challenges that may arise with AI use. Training programs in medical schools, residency programs, and CME sessions should emphasize the responsible use of AI and ethical decision making while developing a culture of ethical awareness in the healthcare community.
Emotions and the ethical use of AI in healthcare
The use of AI in healthcare is not merely a technological shift but one that deeply intertwines with the realm of human emotions. Ethical AI implementation is crucial to mitigate potential harm and uphold patient trust. One significant area of concern is the impact AI may have on patient anxiety or fear. The introduction of AI-powered diagnostic tools, for instance, could lead to increased patient distress if not communicated with sensitivity and empathy.16 The design of these systems must account for emotional nuances, providing explanations that patients can comprehend and addressing anxieties in a supportive manner.17
Moreover, ensuring equity and fairness in AI algorithms is an ethical imperative that directly influences emotional well-being. If these systems perpetuate biases along racial, gender, or socioeconomic lines, the consequences can be profound. Marginalized patients may experience heightened stress, mistrust, and a sense of dehumanization if they perceive the healthcare system as biased against them.16 Rigorous testing for bias and continuous monitoring of AI performance is crucial, along with fostering practitioner awareness of potential algorithm shortcomings.17
Preserving the empathetic human connection in an AI-driven healthcare landscape is paramount. It is essential to maintain a clear distinction between the capabilities of AI and the irreplaceable role of human healthcare practitioners in addressing emotional needs.18 AI can be leveraged to streamline tasks, allowing providers to establish deeper therapeutic relationships with their patients.16 By fostering collaboration between human empathy and AI efficiency, healthcare can not only become more efficient but also more emotionally resonant—creating a system that attends to the whole person rather than just their medical data
As AI continues to transform the healthcare landscape, it is vital to navigate this phase with a keen focus on ethics. Addressing biases in algorithms, obtaining informed consent from patients, and deploying AI technologies responsibly are crucial steps toward ensuring that the benefits of AI in healthcare are realized without compromising fundamental ethical principles. The healthcare industry can use the full potential of AI to improve patient outcomes while protecting the values of medicine by actively engaging in ethical considerations.
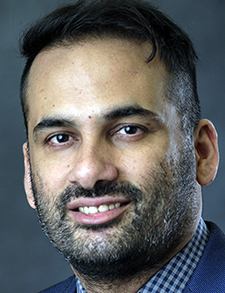
Dr. Dhillon
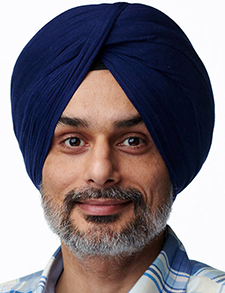
Dr. Grewal
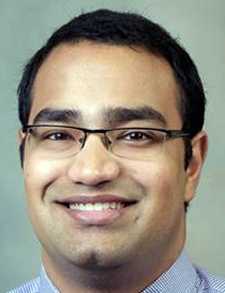
Dr. Buddhavarapu
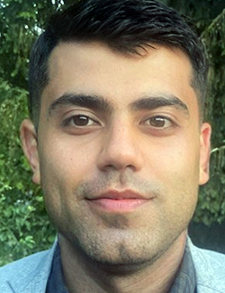
Mr. Virmani
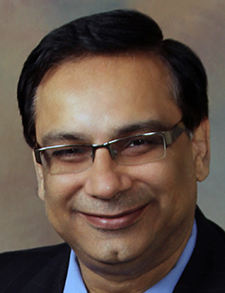
Dr. Surani
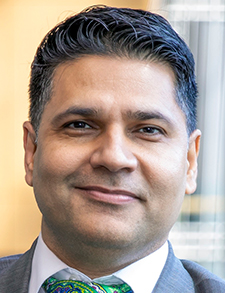
Dr. Kashyap
Dr. Dhillon is the associate medical director of the Adfinitas inpatient hospital team at the University of Maryland Baltimore Washington Medical Center in Glen Burnie, Md., and adjunct assistant professor of medicine at the University of Maryland School of Medicine in Baltimore. Dr. Grewal is a radiologist and an assistant professor at Florida State University College of Medicine in Pensacola, Fla. Dr. Buddhavarapu is a hospitalist at Banner Baywood Medicine Center, Banner Health, in Mesa, Ariz., Mr. Virmani is a senior cloud-data architect at Google. Dr. Surani is an adjunct clinical professor of medicine and pharmacology at Texas A&M University in Corpus Christi, Texas. Dr. Kashyap is a medical director of research at WellSpan Health, in York, Pa., and assistant professor of anesthesiology at Mayo Clinic, in Rochester, Minn.
References
- Grewal H, Dhillon G, et al. Radiology gets chatty: the ChatGPT saga unfolds. Cureus. 2023;15(6):e40135. doi: 10.7759/cureus.40135.
- Farhud DD, Zokaei S. Ethical issues of artificial intelligence in medicine and healthcare. Iran J Public Health. 2021;50(11):i-v. doi: 10.18502/ijph.v50i11.7600.
- Yoon JH, Pinsky MR, Clermont G. Artificial intelligence in critical care medicine. Crit Care. 2022;26(1):75. doi.org/10.1186/s13054-022-03915-3.
- Bohr A, Memarzadeh K. The rise of artificial intelligence in healthcare applications. Artificial Intelligence in Healthcare. 2020:25-60. doi: 10.1016/B978-0-12-818438-7.00002-2.
- Dhillon G, Grewal H, et al. Gender inclusive care toolkit for hospitals. Lancet Reg Health Am. 2023;26:100583. doi: 10.1016/j.lana.2023.100583.
- Riley WJ. Health disparities: gaps in access, quality and affordability of medical care. Trans Am Clin Climatol Assoc. 2012;123:167-72; discussion 172-4.
- du Toit C, Tran TQB, et al. Survey and evaluation of hypertension machine learning research. J Am Heart Assoc. 2023;12(9):e027896. doi: 10.1161/JAHA.122.027896.
- Dellinger EP, Pellegrini CA, Gallagher TH. The aging physician and the medical profession: a review. JAMA Surg. 2017;152(10):967-71.
- Varelius J. The value of autonomy in medical ethics. Med Health Care Philos. 2006;9(3):377-88.
- Tinetti M, Dindo L, et al. Challenges and strategies in patients’ health priorities-aligned decision-making for older adults with multiple chronic conditions. PLoS One. 2019;14(6):e0218249. doi: 10.1371/journal.pone.0218249.
- Fritsch SJ, Blankenheim A, et al. Attitudes and perception of artificial intelligence in healthcare: A cross-sectional survey among patients. Digit Health. 2022;8:20552076221116772. doi: 10.1177/20552076221116772.
- Vokinger KN, Gasser U. Regulating AI in medicine in the United States and Europe. Nat Mach Intell. 2021;3(9):738-9.
- Verma RK, Dhillon G, et al. Artificial intelligence in sleep medicine: present and future. World J Clin Cases. 20236;11(34):8106-10.
- Stern AD, Goldfarb A, et al. AI insurance: how liability insurance can drive the responsible adoption of artificial intelligence in health care. NEJM Catalyst. 2022.3(4). doi: 10.1056/CAT.21.0242.
- Hatem R, Simmons B, Thornton JE. A Call to address AI “hallucinations” and how healthcare professionals can mitigate their risks. Cureus. 2023;15(9):e44720. doi: 10.7759/cureus.44720.
- Char DS, Shah NH, Magnus D. Implementing machine learning in health care — addressing ethical challenges. N Engl J Med. 2018;378(11), 981-3.
- Obermeyer Z, Powers B, et al. Dissecting racial bias in an algorithm used to manage the health of populations. Science. 2019;366(6464):447-53.
- Mohanasundari SK, Kalpana M, et al. Can artificial intelligence replace the unique nursing role? Cureus. 2023;15(12):e51150. doi: 10.7759/cureus.51150.